ISU researcher John Kalivas to use big data to refine cutting-edge chemical analysis methods
October 1, 2019
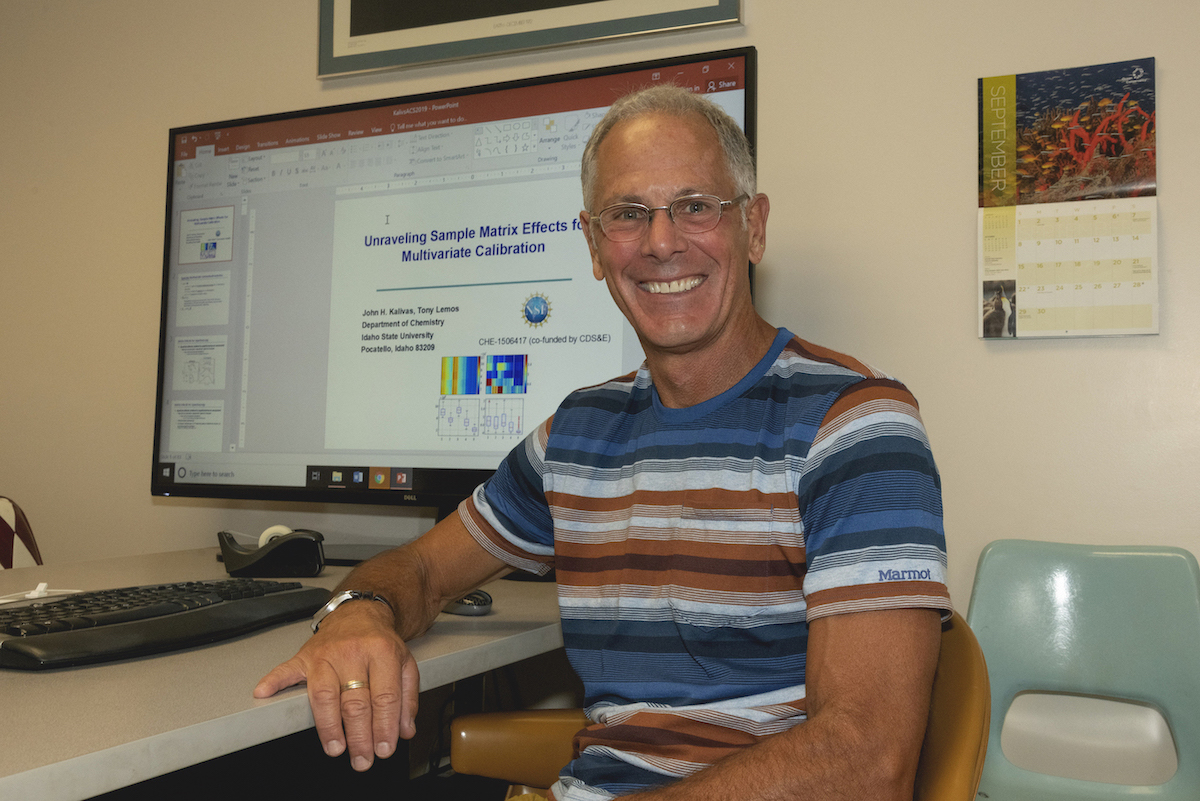
POCATELLO – Imagine a time when a consumer could take out their cell phone, which is now equipped with a small spectrometer, and first stopping to fuel their car, could zap the gas to check its octane content to make sure they’re getting what their paying for.
Then, while shopping, the consumer could use their phone’s spectrometer to check the sugar content of apples before buying them. Once home with the groceries, a smart phone could be used to check the purity of the olive oil they’ve bought and to make sure that pills from the pharmacy contain the proper amount of active pharmaceutical ingredient.
This scenario isn’t possible yet, but Idaho State University chemistry Professor John Kalivas is working on mathematical formulas and algorithms that may one day make this possible by using artificial intelligence and machine learning to harvest big data to enable spectrometers to be more broadly and accurately used.
Kalivas is an analytical chemist who, since the late 1970s as a graduate student, and since 1985 as an ISU faculty member, has used “chemometrics” – using math in the same way as artificial intelligence and machine learning does – to build mathematical models relating instrument signals to the physical and chemical properties of samples. Often, he uses spectra, the data collected by spectrometers, to make these models that can predict anything from the boiling point or concentration of a sample, to the authenticity of a commercial product. Spectrometry is the study of interactions between light and matter, and the reactions and measurements of radiation intensity and wavelengths.
“We deal with spectral data, that’s our instrument signal, like infrared spectroscopy, or fluorescence spectroscopy,” Kalivas said. “When samples come in we can measure a spectrum in a second.”
He has developed models generated by computers that can be used to identify the purity of olive oil, the pulp content of trees, the carbon and hydrogen content of soil, and the active pharmaceutical ingredient of medicine tablets – and these are just a few examples. These models make it possible to understand the chemical makeup of things without doing complex laboratory analyses, reducing analysis time and costs.
In the past, Kalivas and the undergraduates working in his laboratory, have created transferable models for using spectrometry to determine characteristics of samples. For example, they could input spectral data, run algorithms to develop the model and determine the pulp content of Douglas Fir trees for a geographical area. That model could then could be transferred to a different location in the West, but the model has to be updated using a few samples from the new geographical area so it would be accurate in the new conditions. In machine learning language, this process is known as transfer learning.
However, now, funded by a new $400,000 National Science Foundation grant that is one of the many NSF grants that Kalivas has received through the years, the ISU professor will use a super computer to mine available data to create models that are accurate, without having to update the models under new conditions. The title of the grant is “Adaptive Learning for Multivariant Calibration with Big Data Attributes” and will use the Extreme Science and Engineering Discovery Environment supercomputer system.
The key word above is “adaptive.” Kalivas and his students will attempt to create algorithms and computational code to build models as needed that adapt to new conditions by “data mining” existing spectral data and seeking accurate matches to new samples. For example, one of the many databases Kalivas will use is a library of 60,000 worldwide soil samples with known reference values such as soil organic content. To test the research, he will use a random soil sample and then search through the soil library to find the best matches to form the model. He’ll then check to see if the random sample is accurately determined by the just formed model. This sounds relatively simple, but it is a difficult mathematical challenge due to the underlying complex chemical and physical nature of samples.
“We hope to do on-the-fly analysis,” Kalivas said. “We will take a spectrum (of a sample) and immediately give an answer. The model we’ve built has to adapt to the particular sample. What we are doing is a totally different approach to what we’ve done in the past and it requires big data so you can zap a sample (with a spectrometer), mine through the data and determine an accurate model.”
The Kalivas lab began working on the grant in August, and will work on it for a total of three years. At least four undergraduate students will work on the project, learning state-of-the-art modeling methods and becoming proficient at performing scientific research. The project will also develop software tools for use in the analytical curriculum at ISU. All developed algorithms will be posted on the Kalivas website, allowing free access to potential users.
####
Idaho State University, a Carnegie-classified doctoral high research activity university and teaching institution founded in 1901, attracts students from around the world to its Idaho campuses. At the main campus in Pocatello, and at locations in Meridian, Idaho Falls and Twin Falls, ISU has nine Colleges, a Graduate School and a Division of Health Sciences that together offer more than 250 certificate and degree programs. More than 12,000 students attend ISU. Idaho State University is the state's designated lead institution in health professions.
Categories: